Researchers at IDEAS-Science Ltd., in collaboration with the AGIgent team, have carried out an experiment by combining deep CNN network train process with MicrobeSim holographic image simulator. This experiment pushes the boundaries of digital holographic microscopy (DHM) by training artificial intelligence on 70,000+ synthetic holograms of particles with various shapes and structures. The MicrobeSim innovative simulator generates single particles ranging from 0.1 µm to 10 µm in size, while CNN network learns the low level principles behind complex refractive phenomenons. The experiment explores the theoretical capabilities of AI-based classification. MicrobeSim goes beyond traditional Mie-based models by accurately mimicking complex microbe structures with internal features, particle attachments, and realistic lens distortion, providing a rich dataset that mirrors real-world holographic imaging.
The comprehensive dataset encompasses two major classes (and 14 subclasses) – cellular (with subclasses: microbial particles such as hyphae, yeasts, bacteria, spores, and viruses) and homogeneous (subclasses: non-living particles like various types of ash, mineral dust, metals, microplastics, salt, oil, and bio-debris). Training the CNN on this detailed dataset not only achieved 100% accuracy in distinguishing microbial particles from non-living dust but also revealed subtle challenges; for instance, bio-debris was misclassified as microplastic in 15% of cases and as mineral dust in 1% of cases.
This pioneering work demonstrates the potential of combining high-fidelity simulations with AI to create adaptable, pre-trained models ready for fine-tuning with real world data. By establishing a robust foundation through simulated holograms, the approach ensures that the network can generalise to recognise previously unseen particles based on their inherent physical and optical properties.
These advances hold significant potential for improving real-time bio-detection in environmental monitoring, disease diagnostics, and biothreat detection, making AI-driven holographic microscopy a promising tool for future scientific and industrial applications.
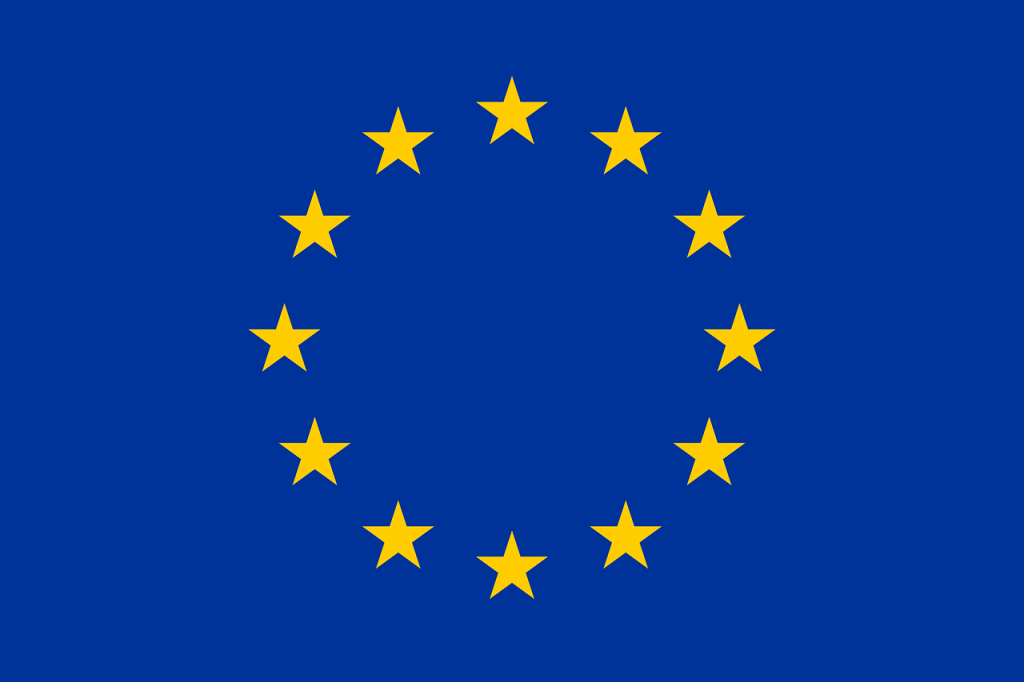
This project has received funding from the European Union’s Horizon 2020 research and innovation programme under grant agreement No 101021723.